A beginner’s guide to AI Edge computing: How it works and its benefits
Discover how edge AI enhances real-time decision-making by processing data locally, improving efficiency, security, and reducing costs across industries.
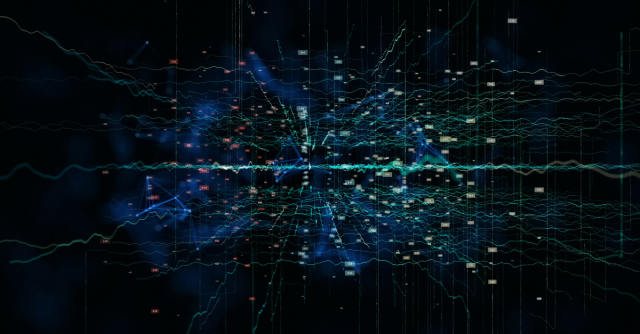
What is Edge AI?
Edge AI refers to deploying artificial intelligence (AI) applications on devices located near the user, at the network edge, rather than relying on centralized cloud infrastructure. This setup allows AI algorithms to be processed directly on local devices, enabling real-time decision-making.
By combining edge computing with AI, businesses address issues of latency and connectivity associated with cloud data centers. Edge AI processes data where it is generated, providing a significant advantage for time-sensitive applications like autonomous vehicles, healthcare monitoring, and industrial automation.
Additionally, processing data locally on the edge reduces the dependency on constant internet connections, enhancing operational efficiency without relying on traditional cloud computing.
Benefits of Edge AI
Edge AI brings several key benefits to organizations:
Real-time data processing
By processing data directly on an edge device, businesses can make instant decisions without delays. This is critical in fields like autonomous vehicles and healthcare, where immediate responses are necessary.
Enhanced data privacy and security
Processing sensitive data locally reduces risks related to transmission to cloud servers. This is especially important for industries like healthcare and finance.
Cost efficiency
By processing data locally, businesses reduce the need for costly cloud resources, which is particularly useful in environments with limited connectivity.
Reduced network strain
Edge AI decreases network bandwidth usage by reducing the amount of data sent to and from centralized systems.
How Edge computing works
Edge computing brings the data processed closer to where it’s generated, significantly reducing latency and enhancing response times. Instead of relying on the cloud or centralized infrastructure, edge computing distributes computing tasks across edge devices and edge servers located near the source of the data collected.
This decentralized approach is especially effective in environments where real-time insights and decision-making are critical, such as in IoT-driven industries, autonomous vehicles, manufacturing, and healthcare.
By processing its data locally, businesses alleviate the strain on network bandwidth. Rather than transferring vast amounts of data back and forth between centralized servers and client devices, edge computing processes data on-site, reducing network congestion and allowing for faster, more efficient analytics. This capability is essential for applications like smart manufacturing, automated supply chains, and remote health monitoring systems, where real-time data processing can improve outcomes and streamline operations.
A key advantage of edge computing is its ability to function independently of constant internet connectivity. In areas with unreliable or limited network access, an edge device can still process and store data locally, making them ideal for remote or off-grid locations like offshore oil rigs, agricultural operations, and remote manufacturing sites.
Once network access is restored, only the necessary data can be transmitted back to a centralized database for further analysis, reducing unnecessary data transmission.
Additionally, edge computing enables organizations to deploy AI models directly on devices, analyze data in real time, and make swift decisions without relying on constant communication with centralized systems.
For example, in a smart factory, edge devices can analyze data generated from connected machinery, identify operational inefficiencies, and automatically adjust parameters in real time. This not only improves operational efficiency but also reduces downtime, leading to greater productivity.
Ultimately, edge computing plays a crucial role in reducing latency, improving data privacy, and speeding up response times, allowing industries to react quickly to changes and optimize their operations. By bringing AI and data processing closer to the network edge, businesses can create a more seamless experience for end users, unlocking the full potential of edge technology.
Edge AI applications
Edge AI’s versatility enables it to drive innovation across a variety of industries, delivering real-time processing and decision-making where speed and accuracy are critical. From enhancing everyday technology to powering advanced systems, using artificial intelligence at the edge is playing a transformative role across sectors:
Facial recognition: One of the most widespread applications of Edge AI is facial recognition, used in smartphones, security systems, and IoT devices. Edge AI enables instantaneous recognition, enhancing security and user experience without the latency of sending data to the cloud for analysis.
In smart buildings and public safety systems, for instance, facial recognition powered by Edge AI can quickly identify authorized personnel, alert security teams in real time, and provide a faster, more secure user experience. This also ensures that sensitive data remains on local devices, reducing the risk of breaches.
Healthcare: In healthcare, Edge AI is revolutionizing patient care by processing medical data in real time. From monitoring vital signs to detecting early warning signs of health anomalies, Edge AI allows medical professionals to act swiftly and provide targeted treatments.
For example, in emergency care, Edge AI devices can monitor data such as heart rate, oxygen levels, and blood pressure, and immediately alert medical staff if something goes wrong, ensuring timely interventions. By keeping sensitive patient data local, Edge AI minimizes the risks associated with transmitting medical information to cloud servers, reducing privacy concerns and ensuring compliance with healthcare regulations like HIPAA.
Autonomous vehicles: Autonomous vehicles rely heavily on Edge AI to process vast amounts of sensor data in real time. Edge AI processes information from cameras, radar, and LIDAR systems to help vehicles navigate, detect obstacles, and make split-second decisions critical for passenger safety.
By processing this data locally within the vehicle, Edge AI eliminates the need for continuous cloud communication, enabling the car to respond in milliseconds. This not only enhances the safety of autonomous driving but also ensures vehicles can operate in areas with limited or no internet connection, making them more reliable in diverse environments.
Smart devices: Edge AI is integral to the operation of smart devices such as smart speakers, thermostats, and cameras, providing real-time interactions and enhancing user experiences. For instance, smart speakers equipped with Edge AI can process voice commands locally, delivering quicker responses to queries without the delay of cloud processing. Similarly, security cameras with Edge AI can instantly detect movement, identify potential threats, and trigger real-time alerts, improving home and business security. By processing this data locally, these devices function more efficiently, even where network bandwidth is limited, contributing to smarter, more responsive homes and workplaces.
Edge AI's ability to process data at the point of collection allows for more immediate and reliable performance across these applications. Whether it’s for safety, convenience, or operational efficiency, Edge AI models are shaping the future of technology across a variety of industries.
Real-time data processing with Edge AI
One of Edge AI’s most valuable features is its ability to deliver real-time data processing. Edge devices can execute machine learning (ML) and deep learning models (DL) directly on-site, allowing for immediate decision-making in mission-critical environments such as autonomous vehicles, industrial automation, and healthcare.
For example, while data processing in the cloud can take a few seconds, data processing at the edge occurs in milliseconds or less. This rapid response time is crucial for applications where even minor delays can impact performance or safety.
By processing collected data locally, Edge AI reduces reliance on network bandwidth, improves operational efficiency, and ensures organizations can react quickly to changing conditions.
Edge AI trends and the future
The Edge AI market is undergoing rapid growth, with projections suggesting it will reach $62.93 billion by 2030. This expansion is driven by the increasing need for real-time data processing and localized decision-making across industries like manufacturing, transportation, and retail. These sectors are adopting Edge AI solutions to streamline operations, enhance operational efficiency, and reduce costs.
Forrester’s Top 10 Trends In Edge Computing And IoT Report is your compass to the latest in edge computing and IoT. Whether you’re a seasoned tech leader or just beginning to explore the potential of the edge, this report provides the insights you need to push boundaries and drive success.
One of the key drivers behind this growth is the deployment of 5G networks, which accelerates data transmission speeds and reduces latency. The integration of 5G with Edge AI technology allows for large-scale, high-speed data collection and processing at the network edge, enabling organizations to analyze data using AI models in real time. This is crucial for applications like autonomous vehicles, smart city initiatives, and industrial IoT, where split-second decision-making is essential.
Additionally, edge-to-edge collaboration is emerging as an important trend. This allows an edge device to communicate directly with another, improving decision-making and processing data across decentralized networks. This trend is particularly relevant in healthcare, smart homes, and manufacturing, where real-time data processing can optimize workflows and enhance user experiences.
Another significant development is the rise of AI-powered video surveillance and energy management systems. These systems leverage Edge AI to perform real-time analysis of video footage and energy data, respectively. For example, video surveillance systems use machine vision to improve public safety, while energy management systems utilize artificial intelligence algorithms to optimize resource distribution in smart grids.
As Edge AI continues to evolve, its integration with IoT devices will drive even greater innovation across industries. This includes applications like smart wearables, autonomous drones, and industrial robots, which will increasingly rely on localized AI processing to operate more autonomously and efficiently.
Edge AI security and privacy
Processing data locally at the network edge offers significant security advantages, minimizing the need to transmit sensitive data across networks. Edge devices are equipped with robust security measures like encrypted authentication, secure data storage, and data integrity auditing.
Reducing reliance on centralized cloud systems lowers the risk of hacking or data interception during data transmission. Local data processing ensures information remains secure within the device, making it easier to comply with privacy regulations like HIPAA and GDPR.
Edge AI and cloud computing
While Edge AI operates locally, cloud computing remains a valuable partner in an edge AI deployment. The cloud provides the necessary infrastructure for scalable storage, cost efficiency, and collaboration. For example, AI algorithms can be trained using the extensive computing power of cloud data centers and then deployed on an edge device for real-time execution, allowing businesses to take advantage of both the edge and the cloud's scalability.
By running AI models on the edge, organizations minimize the need for constant data transmission between edge devices and the cloud, reducing both network bandwidth usage and reliance on cloud resources for inference. This approach also helps reduce operational costs by processing data locally, all while maintaining the benefits of cloud-based artificial intelligence training, collaboration, and scalability.
Furthermore, this combined setup enables businesses to optimize the balance between local and cloud-based processing. For instance, deep learning models can be fine-tuned in the cloud, but real-time inference can occur at the network edge, enhancing response times and reducing latency. This hybrid model ensures that businesses can maintain high-performance computing without sacrificing flexibility.
Getting started with Edge AI
Implementing Edge AI can seem complex, but with a clear strategy with the right components and the right partners, businesses can unlock its powerful benefits. Here’s how to get started:
1. Define your use cases
Begin by identifying where Edge AI can offer the most value, as it excels in scenarios requiring real-time data processing and decision-making, such as predictive maintenance in industrial operations, real-time insights in health monitoring, and autonomous vehicle navigation. Defining specific business objectives and pain points will help focus your deployment on the areas that can deliver the highest impact, especially in mission-critical environments.
2. Select appropriate hardware
Choosing the right hardware is key to a successful deployment. Depending on your use case, this may involve selecting IoT devices with built-in AI processors, such as GPUs, ASICs, or servers that can handle more substantial AI workloads.
Flexential colocation and interconnection services provide scalable infrastructure options that can support the hardware needed for AI at the edge, ensuring low latency and high performance. Additionally, selecting hardware capable of high-performance computing capabilities is crucial for handling deep learning models and machine vision applications.
3. Leverage cloud and edge synergy
While Edge AI focuses on local data processing, many solutions benefit from cloud computing integration for tasks like AI model training, data backup, and analytics. This cloud-edge synergy ensures that while critical data is processed at the edge, more complex workloads can still be handled in a cloud data center when necessary. By reshaping your approach to digital infrastrcuture and integrating cloud-based infrastructure with edge technology, businesses can strike a balance between local processing and the scalability of the cloud.
4. Pilot and scale
Start with a small, targeted pilot project to test Edge AI’s performance in your environment. Once the technology has proven its value, you can scale to more applications. Flexential solutions allow businesses to grow their edge infrastructure as their needs expand, providing the flexibility to increase capacity without a significant upfront investment. Whether handling large amounts of data from connected devices or scaling up edge servers to process more data, this approach ensures smooth scalability.
Conclusion
To maximize the potential of Edge AI and edge computing, businesses need secure and reliable infrastructure that can extend applications to the edge. Flexential provides a national colocation footprint, interconnection options, and professional services that enable organizations to improve distributed user experiences by bringing applications closer to the end user.
With over 40 data centers across 19 high-growth markets and access to 300+ on-net carriers, the Flexential fleet of strategically positioned data centers are designed to reduce network latency and deliver scalable, repeatable deployments.
Whether you’re deploying IoT devices, running AI applications, or needing fast, reliable data processing, Flexential edge computing solutions can help you create a robust network that supports the demands of a distributed user base. Explore Flexential data center, edge computing, and colocation services to enhance your AI infrastructure and deliver better outcomes for your business.